Data engineering is the backbone of modern, data-driven organisations, playing a pivotal role in developing, deploying, and maintaining robust data pipelines and infrastructure.
Common Methods and Algorithms
- ETL (Extract, Transform, Load): ETL is a core component of data engineering services, enabling the seamless movement of data from multiple sources into a unified, analysis-ready format. Modern ETL pipelines are built to be scalable and adaptable, processing vast amounts of data with low latency. Some providers also implement ELT (Extract, Load, Transform), which allows for faster data movement when transformations can be deferred.
- Batch Processing vs. Stream Processing: The choice between batch and stream processing depends on specific business needs. For applications that require immediate insights, such as fraud detection or stock market analysis, stream processing is indispensable. On the other hand, batch processing is more suitable for less time-sensitive tasks like periodic reporting. Consultants evaluate your business requirements and implement the appropriate processing approach—or a hybrid model—to optimise performance.
- Distributed Computing: When dealing with massive data volumes, distributed computing frameworks like Apache Hadoop, Spark, and Flink are essential. These technologies enable the parallel processing of large datasets, ensuring faster insights and better system scalability. Cloud-based data engineering solutions leverage these frameworks to support large-scale data operations while maintaining efficiency.
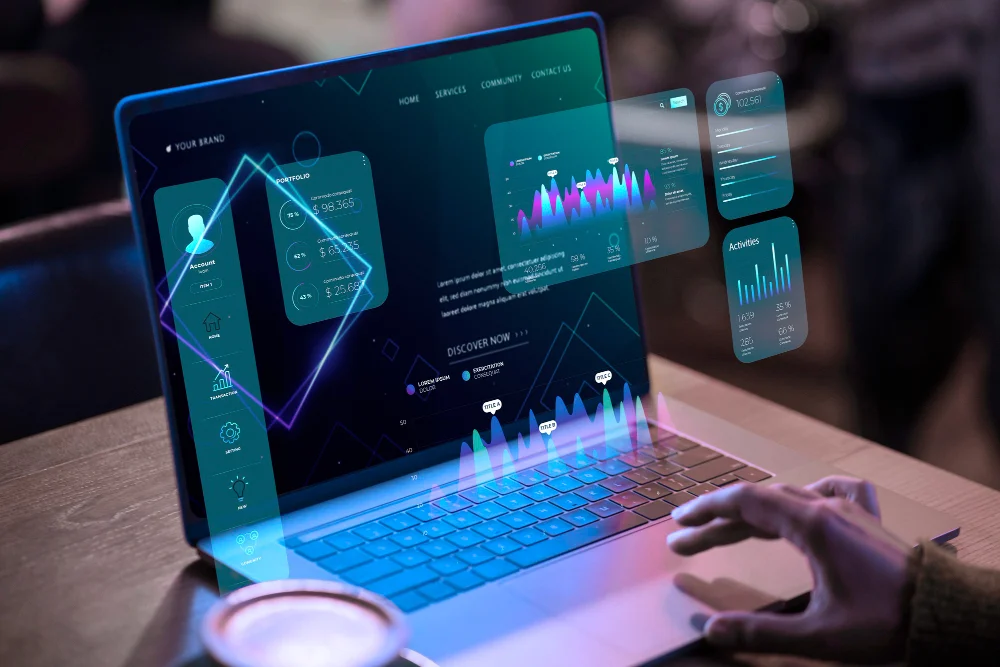
Benefits of Data Engineering
Improved Data Quality
Enhanced Scalability
Faster Time-to-Insight
Cost Optimization
Data-Driven Innovation
Common Methods and Algorithms
ETL (Extract, Transform, Load)
ETL is a core component of data engineering services, enabling the seamless movement of data from multiple sources into a unified, analysis-ready format. Modern ETL pipelines are built to be scalable and adaptable, processing vast amounts of data with low latency. Some providers also implement ELT (Extract, Load, Transform), which allows for faster data movement when transformations can be deferred.
Batch Processing vs. Stream Processing
The choice between batch and stream processing depends on specific business needs. For applications that require immediate insights, such as fraud detection or stock market analysis, stream processing is indispensable. On the other hand, batch processing is more suitable for less time-sensitive tasks like periodic reporting. Consultants evaluate your business requirements and implement the appropriate processing approach—or a hybrid model—to optimise performance.
Distributed Computing
When dealing with massive data volumes, distributed computing frameworks like Apache Hadoop, Spark, and Flink are essential. These technologies enable the parallel processing of large datasets, ensuring faster insights and better system scalability. Cloud-based data engineering solutions leverage these frameworks to support large-scale data operations while maintaining efficiency.
How Does Data Engineering Work?
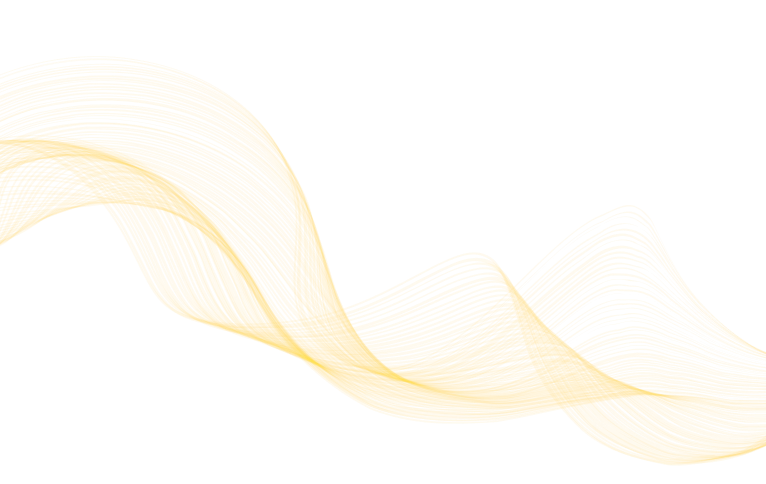
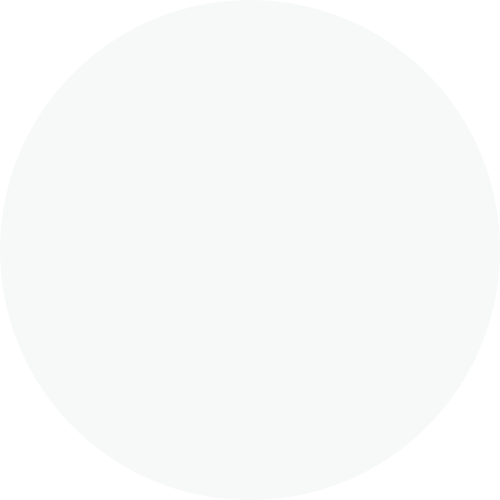
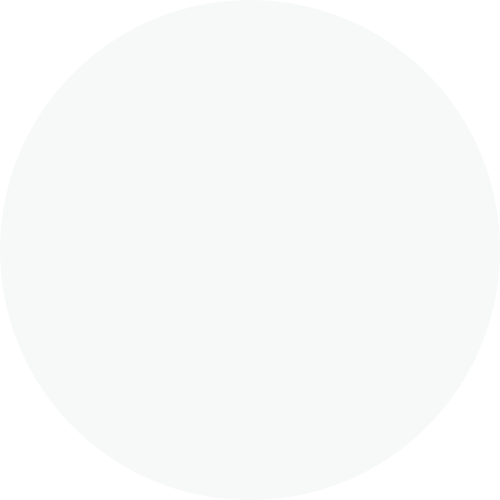
Data Collection and Ingestion
Data Storage and Management
Data Processing and Transformation
Data Analysis and Visualisation
Get Your Free Audit Now!
Get A Quote!
Fill out our contact form, and we will get in touch with you with a quote as soon as we can!
Frequently asked questions
What are data engineering services?
What services do data engineering companies provide?
Why are data engineering solutions important for businesses?
What does a data engineer consultant do?
How can data engineering consultants help optimise data workflows?
How do these solutions improve data quality?
What technologies are commonly used in data engineering?
Can data engineering services be customised for different businesses?
How does ETL contribute to data engineering?
How do I choose the right data engineering company for my business?
Client Testimonials
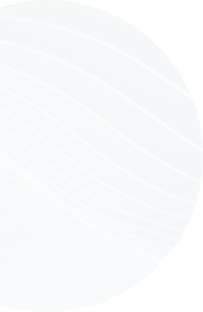